In today’s digital landscape, artificial intelligence (AI) is a powerful tool for improving efficiency, streamlining processes, and delivering new insights. However, AI is not a universal solution; its value depends on context. Deciding when to use AI and when to opt for traditional methods is crucial to avoid wasted resources and ineffective outcomes. Here’s a structured guide to help you navigate when to deploy AI and when it may not fit best.
When to Use AI
Step 1: Assessing Repetitive Tasks – Ideal for AI Automation
When to Use AI
If your team is bogged down by high-volume, repetitive tasks, AI can offer substantial relief by automating these workflows. This includes data processing, customer service responses, and content categorization—tasks that demand high accuracy and consistency but are repetitive for human employees.
Why It Works
AI excels at handling tasks that require consistency without variation, significantly reducing human error and speeding up results.
Example
Customer service teams often implement AI chatbots to handle frequent queries, allowing them to focus on more complex customer interactions.
Step 2: Data-Heavy Analysis – AI for Uncovering Patterns
When to Use AI
AI-powered analytics is invaluable when your goals involve analyzing massive data sets to identify patterns, trends, or actionable insights. AI models can process and interpret data faster than human analysts, identifying relationships that may not be visible otherwise.
Why It Works
AI algorithms are designed for large-scale data interpretation, processing complex data more quickly and efficiently than traditional analysis methods.
Example
Financial institutions use AI to analyze customer spending behaviors, helping them tailor offerings and detect unusual activity.
Step 3: Predictive Modeling – AI for Future Forecasting
When to Use AI
For projects requiring predictions, AI-based predictive modeling can forecast future trends based on historical data. This is particularly valuable for demand forecasting, customer retention analysis, and equipment maintenance predictions.
Why It Works
Machine learning algorithms analyze past data to predict future outcomes, offering businesses a proactive approach to strategy and decision-making.
Example
Retail companies use AI-driven demand forecasting to optimize inventory, reducing waste and increasing efficiency.
Step 4: Personalization and Customer Insights
When to Use AI
AI is an effective tool for personalizing user experiences, making it ideal for recommendations, content customization, and targeted advertising.
Why It Works
AI tailors customer experiences by leveraging data on user behavior, preferences, and previous interactions, enhancing customer satisfaction and engagement.
Example
Streaming platforms like Netflix use AI to suggest content tailored to individual viewing habits, keeping viewers engaged.
Step 5: Complex Problem Solving and Research
When to Use AI
In research and development, AI can simulate and test solutions, especially for complex problems in fields like scientific research, engineering, medical and pharmaceuticals. AI’s ability to run thousands of simulations rapidly helps teams develop solutions faster.
Why It Works
AI-driven simulations provide a quick, cost-effective way to test ideas without the risks or expenses of physical testing.
Example
Pharmaceutical companies use AI in drug discovery to predict how compounds will interact, speeding up the time it takes to bring new medications to market.
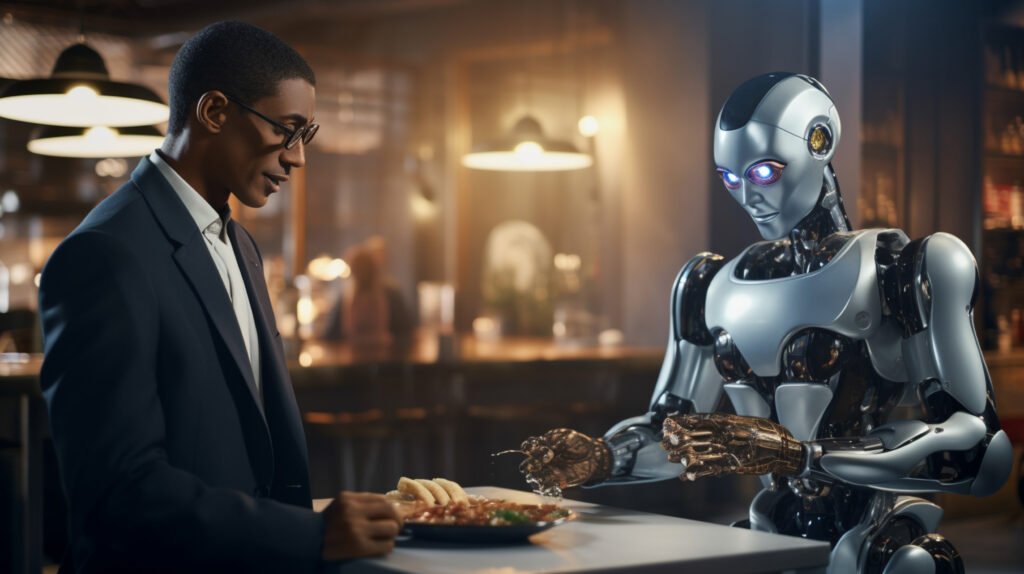
When NOT to Use AI
Step 6: Small-Scale Problems or Projects
Why Not to Use AI
Small projects with manageable scope may not justify the investment in AI. For tasks that are easily resolved by manual methods, implementing AI can be unnecessary and overly complex.
Alternative Approach
Simple automation tools or manual methods can often handle small tasks more cost-effectively.
Example
A small business processing limited orders might manage inventory with manual checks rather than investing in AI.
Step 7: Insufficient Data Quality or Volume
Why Not to Use AI
AI relies on vast, high-quality data sets to function accurately. If data is incomplete, outdated, or poorly structured, AI output may be inaccurate or biased.
Alternative Approach
Improve your data quality through cleansing and structuring before considering AI, or rely on manual analysis for more nuanced insights.
Example
Using AI for customer analysis with incomplete data will result in unreliable insights, potentially leading to poor decisions.
Step 8: Projects Requiring Human Judgment
Why Not to Use AI
Certain tasks require empathy, ethical judgment, or deep context—areas where AI may not be reliable. This includes roles like hiring, performance evaluations, and negotiations, where human judgment is crucial.
Alternative Approach
Human oversight and decision-making are essential for tasks where social nuances, empathy, or ethics are central.
Example
In recruitment, AI can assist in screening résumés but may miss subtleties important for assessing cultural fit, which are better evaluated by humans.
Step 9: Privacy-Sensitive and Regulatory Environments
Why Not to Use AI
Implementing AI in settings with strict privacy requirements, like legal or medical fields, may pose compliance risks. AI algorithms can sometimes overlook data privacy, leading to potential breaches.
Alternative Approach
In privacy-sensitive fields, use human-led processes to ensure compliance with industry regulations and mitigate the risk of data breaches.
Example
Healthcare providers handling sensitive patient data often rely on secure, human-driven methods for processing and analyzing information.
Step 10: Short-Term or Experimental Projects
Why Not to Use AI
AI implementation requires a significant time and cost investment. For projects with short-term goals, the setup and maintenance of AI may not yield a worthwhile return.
Alternative Approach
For temporary needs, consider simpler tools or manual solutions that don’t require extensive setup.
Example
A short-term social media campaign might be better managed by existing analytics tools rather than a custom AI-driven solution.
Key Considerations Before Using AI
Before implementing AI, consider these questions to ensure it’s the right choice:
- Is the task repetitive or data-heavy? If yes, AI can likely add value.
- Do you have high-quality data? If not, focus on improving data quality first.
- Is human judgment essential? If yes, AI may not be appropriate.
- Is this a long-term project? If not, simpler methods may be more cost-effective.
- Are there privacy concerns? AI may introduce risks in privacy-sensitive environments.
Benefits of Strategic AI Use
When used responsibly, AI offers:
- Increased Efficiency: Automating routine tasks improves productivity and reduces manual errors.
- Data-Driven Decisions: AI uncovers actionable insights, enhancing strategy and decision-making.
- Improved Customer Experience: Personalization driven by AI improves user engagement and satisfaction.
- Cost Savings: By automating processes and minimizing resource use, AI reduces operational costs.
Final Thoughts
AI can be a game-changer when applied thoughtfully. By understanding where AI shines and where it may fall short, businesses can achieve a balance that leverages AI’s strengths while avoiding common pitfalls. In a world increasingly driven by data and technology, the key is knowing when AI will enhance processes and when human touch and traditional methods still hold the advantage. Use this step-by-step guide to navigate AI implementation with confidence, maximizing its benefits and avoiding unnecessary investments.